The ubiquitous Internet of Things (IoT) system is a key component of future 6G networks to realize a fully connected world. Extensive efforts have been made to provide on-demand traffic scheduling in IoT networks through machine learning algorithms. However, the current learning approaches are hindered by the heterogeneous information in ubiquitous IoT systems since the data are collected from different domains (e.g., space, air, ground, and ocean). To uncover the complete picture of ubiquitous IoT, this article presents a novel federated imitation learning framework for traffic prediction without compromising privacy. This framework contains a knowledge-sharing module to imitate the status of cross-domain models. After that, we design a distributed resource allocation algorithm, where the IoT devices cooperatively make association decisions using matching theory. Simulation results reveal that our proposed approach outperforms state-of-the-art federated transfer learning and achieves desirable traffic scheduling performance in a cross-domain environment.
Federated Imitation Learning: A Cross-Domain Knowledge Sharing Framework for Traffic Scheduling in 6G Ubiquitous IoT
Additional Info
Source: https://ieeexplore.ieee.org/stamp/stamp.jsp?arnumber=9606808&casa_token=-fIP15fZtX4AAAAA:WJiW6zqK5Hk89htl9S3iyu9ZGsUw4U4TCebLBwHqY01LgheOoxSNIkZsu7AFHjWS4_GGw_l56A
Attachment: Download Attachment
About the Author: hamdan
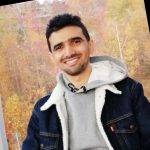
Mohammed Hamdan received his master degree from the Faculty of Computer Science at King Abdulaziz University, Saudi Arabia in March 2018.
He is a Ph.D. student at L'École de Technologie supérieure (ETS) and he has joined Synchromedia since September 2018.
His main research interests are Machine Learning and Image Processing (Pattern Recognition) in the fields of Historical Document image Analysis and Natural Language Processing.
"Knowledge, experience, and expertise don’t have any limit".
Leave A Comment