Classifying document images is a challenging problem that is confronted by many obstacles; specifically, the pivotal need of hand-designed features and the scarcity of labeled data. In this paper, a new approach for classifying document images, based on the availability of footnotes in them, is presented. Our proposed approach depends mainly on a Deep Belief Network (DBN) that consists of two phases, unsupervised pre-training and supervised fine-tuning. The main advantage of using this approach is its capability to automatically engineer the best features to be extracted from a raw document image for the sake of generating an efficient representation of it. This feature learning approach takes advantage of the vast amount of available unlabeled data and employs it with the limited number of labeled data. The obtained results show that the proposed approach provides an effective document images classification framework with a highly reliable performance.
Feature learning for footnote-based document image classification
Additional Info
Source: https://link.springer.com/chapter/10.1007/978-3-319-59876-5_71
About the Author: sherif abuelwafa
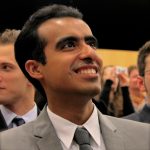
Sherif holds a Ph.D. in machine learning from ÉTS, University of Quebec. His research focuses on encountering the problem of limited availability of labeled data through self-supervised and unsupervised representation learning techniques. Sherif holds a master’s degree in embedded systems design from University of Lugano, Switzerland, where his research focused on designing smart and efficient embedded systems for a wide range of applications. Additionally, he holds a B.Sc. in electronics and telecommunications engineering from Alexandria University, Egypt.
Leave A Comment