Most cities in the world promote bike-sharing services to encourage people to decrease carbon exhausting and to enhance their health. However, it is a big challenge for a bike-sharing service supplying corporation to re-balance bikes efficiently among different bike-sharing dockers without a forecasting ability. For solving this problem, we contribute two new approaches based on standard Long short-term memory (LSTM), which can not only take advantages of multi features inputs and multi-time steps outputs to improve the accuracy of predicting available bikes in one-time step, but also can forecast the number of bikes in multi-time steps. These approaches will help the bike-sharing agencies to make a better decision to distribute their bikes to each docker efficiently. The experimental results confirmed that our multi-feature and multi-time steps models outperform the standard LSTM model.
Multi features and multi-time steps LSTM based methodology for bike sharing availability prediction
Additional Info
Source: https://www.sciencedirect.com/science/article/pii/S187705091930969X
Attachment: Download Attachment
About the Author: Xu Liu
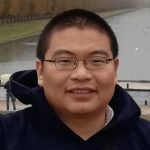
Brain-inspired computing
Deep learning
Machine learning
Embedded system
Image processing
Leave A Comment