Latest Publications
Computing on Wheels: A Deep Reinforcement Learning-Based Approach
Future generation vehicles equipped with modern technologies will impose unprecedented computational demand due to the wide adoption of compute-intensive services with stringent latency requirements. The computational capacity of the next generation vehicular networks can be enhanced by incorporating vehicular edge […]
Energy-aware Control Of UAV-based Wireless Service Provisioning
Unmanned aerial vehicle (UAV)-assisted communications have several promising advantages, such as the ability to facilitate on-demand deployment, high flexibility in network reconfiguration, and high chance of having line-of-sight (LoS) communication links. In this paper, we aim to optimize the UAV […]
Deep Reinforcement Learning for URLLC in 5G Mission-Critical Cloud Robotic Application
In this paper, we investigate the problem of robot swarm control in 5G mission-critical robotic applications, i.e., in an automated grid-based warehouse scenario. Such application requires both the kinematic energy consumption of the robots and the ultra-reliable and low latency […]
Deep Q-Learning for Joint Server Selection, Offloading, and Handover in Multi-access Edge Computing
In this paper, we propose a deep reinforcement learning (DRL) based approach to solving the problem of joint server selection, task offloading and handover in a multi-access edge computing (MEC) wireless network. The 5G networks tend to have a large […]
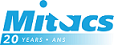










